Bridgestone Tire Sales Prediction
In a project focused on enhancing inventory planning for Bridgestone Americas, a robust forecasting model was developed to improve tire sales predictions. Over three years of transaction-level data served as the foundation for an AutoRegressive Integrated Moving Average (ARIMA) model, designed to capture trends, seasonality, and noise in the data.
Minwoo Sohn
4/28/20232 min read
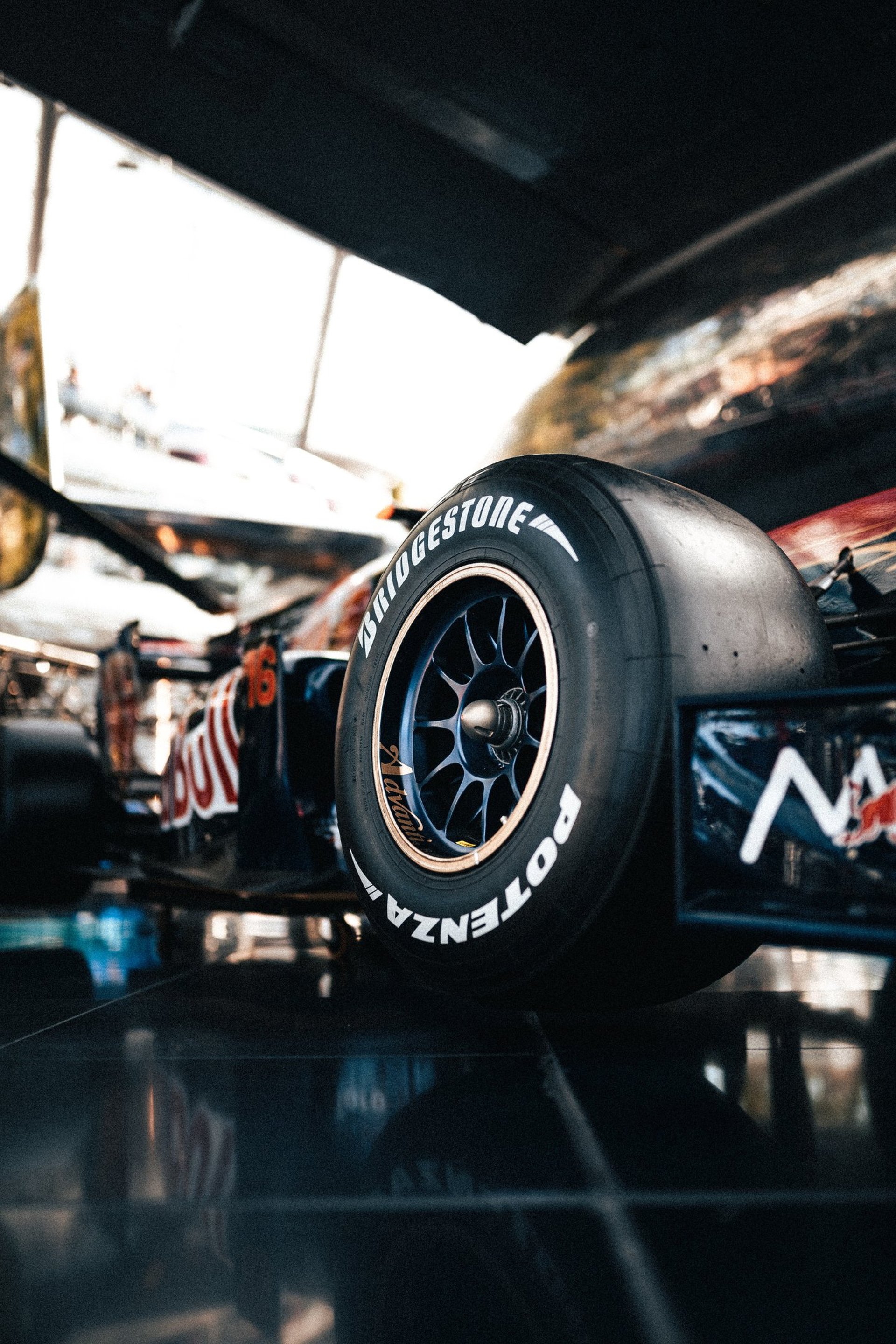
Bridgestone Americas specializes in manufacturing and selling a wide range of tires across the Americas. Accurate inventory planning is crucial for meeting customer demands, especially during high-sales months. In October 2018, the Supply Chain team faced a critical inventory planning phase for tire sales, particularly with the upcoming high-sales month of November. The existing rolling-average methodology lacked predictive accuracy due to its inability to account for trends and seasonality. Our objective was to develop a robust forecasting model using over 3 years of transaction-level sales data.
Data Processing
We initially encountered a 43 GB dataset that was too large to handle. By filtering out non-tire products and low-selling articles, we reduced the data size to under 1 GB, making it more manageable for analysis. This data preparation set the stage for a thorough exploratory data analysis.
Data Exploration
In the data exploration phase, we dug deep into the nuances of tire sales, examining data at individual, store, and vehicle levels. We assessed monthly sales across vehicle manufacturers and evaluated the effect of marketing email opt-ins on customer engagement. We also scrutinized regional sales data to uncover location-specific patterns. Among the categories of tires, we considered were Passenger Tires, Light Truck Tires, Motorcycles, and Road Hazard. Our visual tools included a correlation heatmap and a visualization to identify data gaps. Additional tables and charts provided a well-rounded understanding, paving the way for effective modeling. The insights gained will be leveraged to enhance the sophistication of our future forecasting models.
Modeling
The AutoRegressive Integrated Moving Average (ARIMA) model was our best choice for time-series forecasting. ARIMA excels at capturing various aspects of time-series data, such as trends, seasonality, and noise, through its p, d, and q parameters. In our approach, we utilized the auto_arima function to automatically identify optimal (p, d, q) parameters for each of the 435 articles in the dataset. While the model worked well for 239 articles, it faced issues with 196 others, often producing non-meaningful zero values for p and q. To remedy this, log transformations were applied, successfully modeling an additional 105 articles. The remaining 91 articles had consistent issues, such as data gaps and upward trends. To address these, we used a two-month sales average and a pivot-point strategy, focusing on recent data trends to model most of our dataset successfully.
Evaluation
Our model's performance is assessed via its Weighted Mean Absolute Percentage Error (WMAPE) across all tire articles. Our best model achieved an 18% WMAPE score, significantly improving forecast accuracy.
Location
Nashville, TN